Image Inpainting
The ability of image inpainting to fill in missing image data is useful for many applications. This includes restoration of damaged photographs or image editing (e.g. Photoshop, GIMP, …). In this example, the campus of Saarland University has been masked and is replaced by a lake, fusing the remaining woods convincingly with the new image content.
Our example relies on stable diffusion, which is mostly known as a representative for so-called text-to-image models. These neural networks take a text prompt as an input and output an image. Their reconstruction relies on a model obtained from large amounts of training data and an additional source of randomness.
For inpainting, these models can be multimodal, i.e. a masked input image and a text prompt can be combined. In this case, the positive prompt was “a beautiful lake in the woods” combined with the negative prompt “buildings”.
Interested readers can even try this themselves online, linkable web implementations exist, e.g.: SDXL Inpainting
Original Image
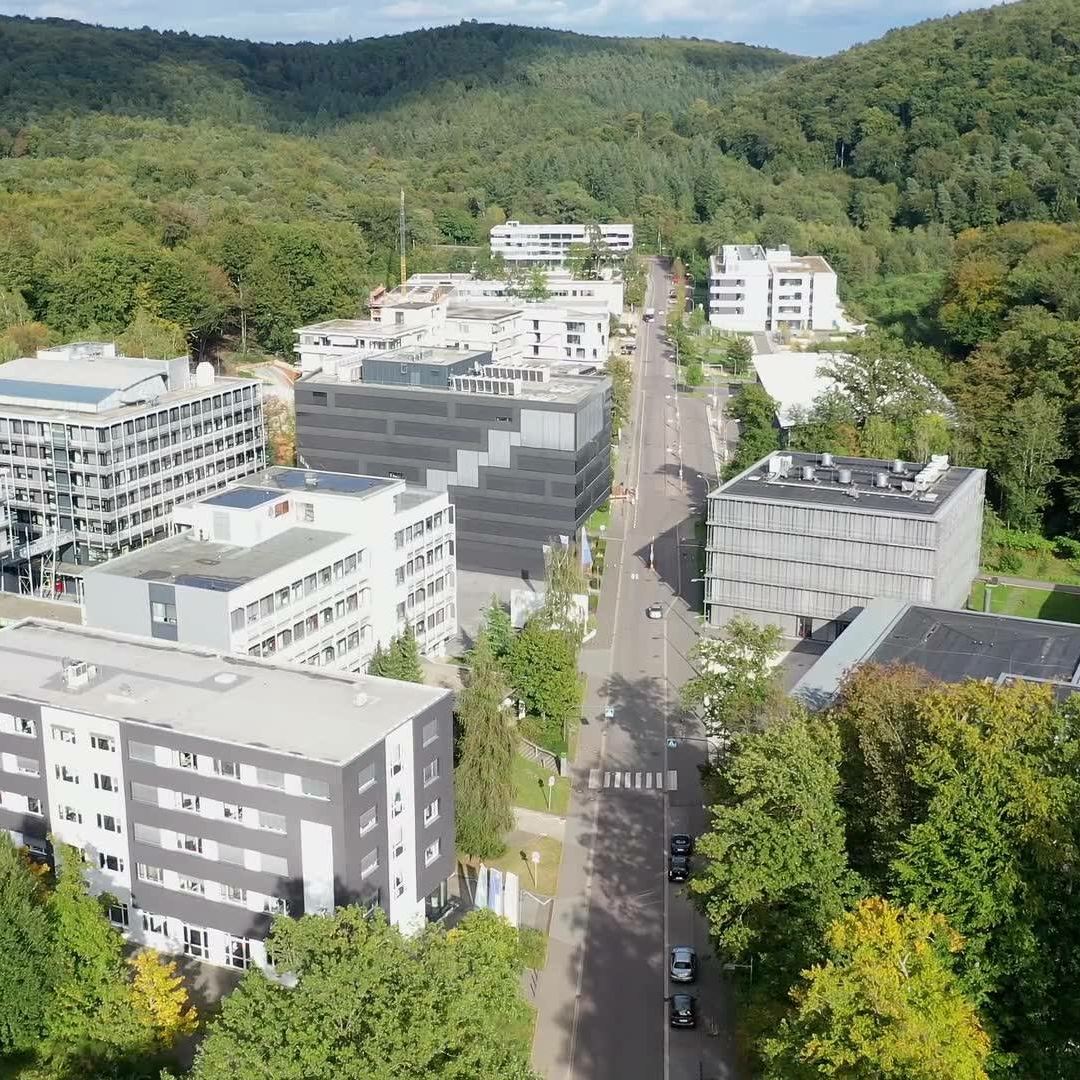
Masked Image
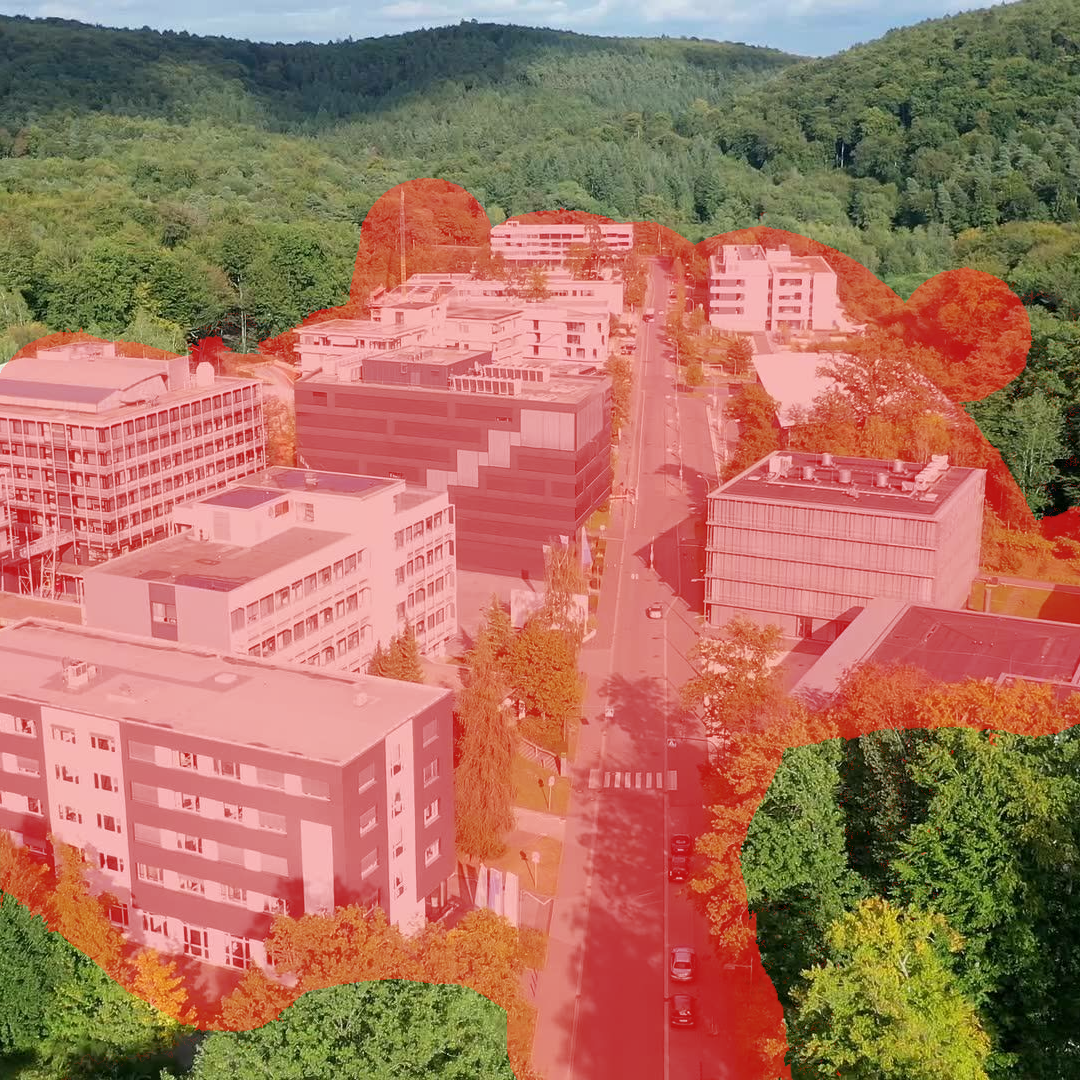
Inpainted Image
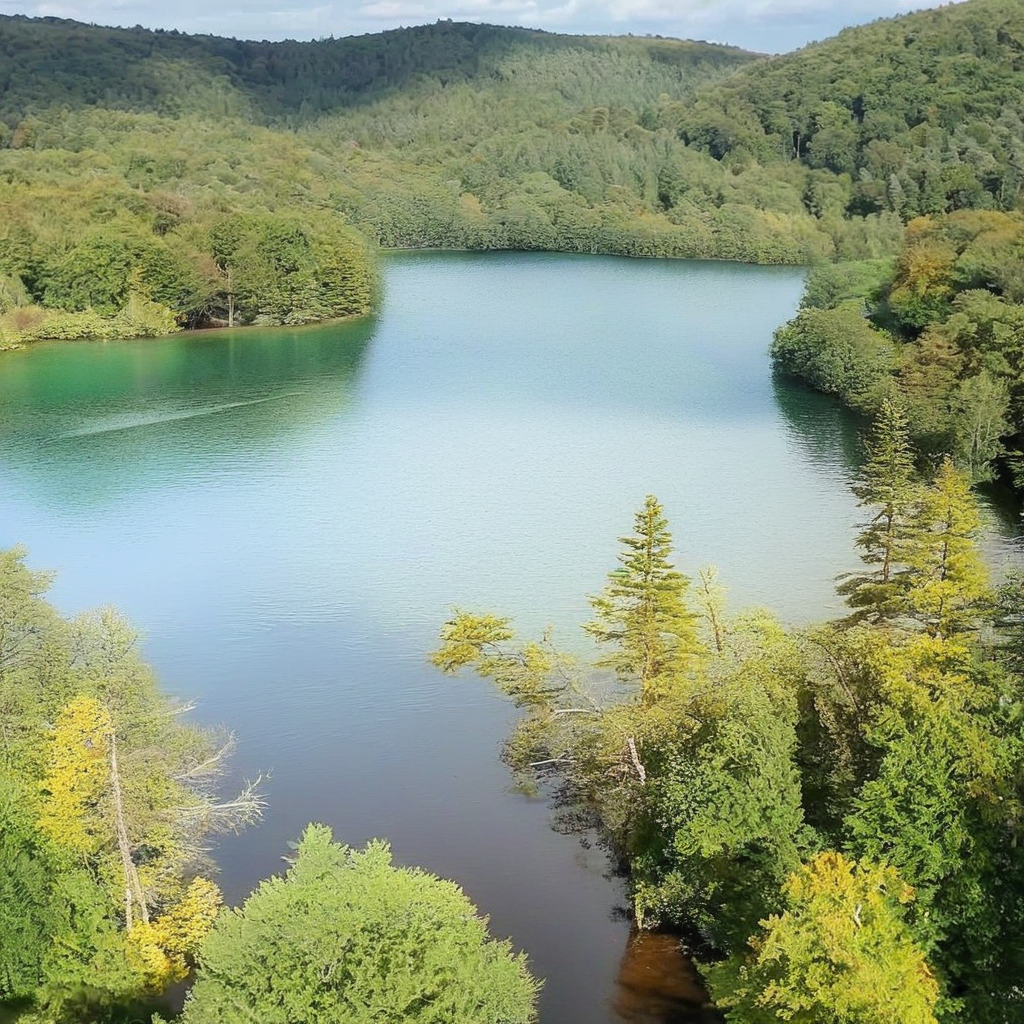
Inpainting from Sparse Data
A deep learning approach has selected 15% of the original pixels from an image showing the campus of Saarland University. Inpainting allows a convincing reconstruction from this very sparse image representation. The reconstruction does not require a neural network, but relies on a filter inspired by physics: It relies on partial differential equations that describe e.g. heat transfer or concentration equilibration.
In combination with data selection strategies as shown in this example, inpainting can be used for image compression by storing only the selected data. Similar techniques are also used for adaptive sampling, e. g. to improve destructive image acquisition methods like electron microscopy.
These examples have been provided by Karl Schrader (Saarland University, Mathematical Image Analysis Group).
Relevant Publications:
Deep spatial and tonal data optimisation for homogeneous diffusion inpainting: Visit site
Efficient Neural Generation of 4K Masks for Homogeneous Diffusion Inpainting: Visit site
Original Image

Sparse Image Representation

Reconstructed Image

DragGAN
This new AI model uses “Generative Adversarial Networks” or GANs and extends possibilities of digital image processing.
Read more


Lightstage
The Lightstage is a device used to generate and record various lighting conditions and light scenes in a controlled manner. The Saarbrücken Lightstage has a diameter of 3.5 meters, includes around 13,000 LEDs, all of which can be controlled individually, and also boasts 40 high-resolution 6k cameras in cinema quality. The Max Planck researchers will use the technology to investigate fundamental problems in the reconstruction and simulation of photorealistic digital characters and environments.
Head of lab Dr. Marc Habermann (l.) und Prof. Christian Theobalt inside Lightstage. The Lightstage is a device used to generate and record various lighting conditions and light scenes in a controlled manner.


SAUCE Project
Image 1: Lightfield-Camera to capture large scenes (several meter in all directions).
Image 2: Setup in one of the TV studios of Saarländischer Rundfunk
Image 3: Cellist Isabel Gehweiler performs a polyphonic piece of music composed by herself.
Video: “Unfolding”, a demo of lightfield capture and processing



Image Captioning
External language models can help for better image captions.
Source: Fusion Models for Improved Image Captioning

